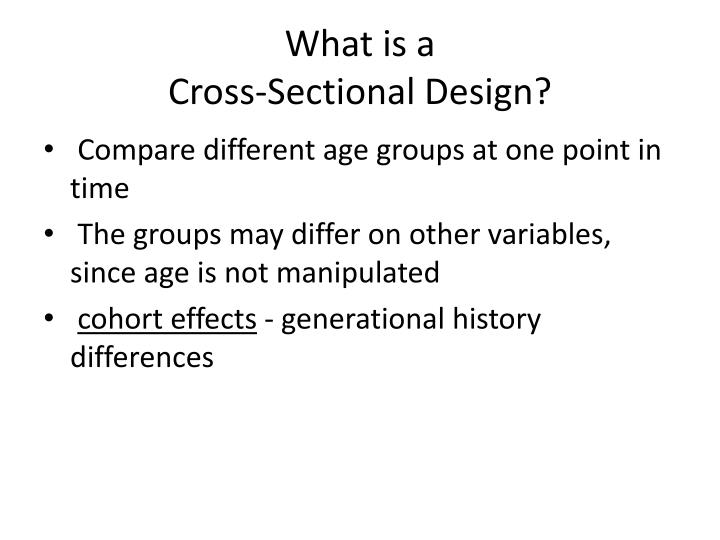
It is a time-saving, cost-effective, and straightforward approach for gathering preliminary data, wherein a researcher collects data at a single point in time (there is no prospective or retrospective follow-up) and observes variables without influencing them. The prevalence of an outcome at a given point in time can be determined in this manner. A cross-sectional study is a type of research that collects data from a group of people at a single point in time to analyze characteristics and relationships.
Strengths of a Cross-sectional Study
Due to some topics’ ethical nature or the need to understand the natural history (i.e., disease or condition), using an observational study design might be the best fit. The primary purposes of observational studies are to describe and examine the distributions of independent (predictor) and dependent (outcome) variables in a population (sample) and analyze the associations between them (Cummings, 2013). Observational studies monitor study participants without providing study interventions. This paper describes the cross-sectional design, examines the strengths and weaknesses, and discusses some methods to report the results. Future articles will focus on other observational methods, the cohort, and case-control designs. One of the main findings of this study was that 94.8% of MUH residents, including smokers, wanted smoke-free policies for MUH.
Does a cross-sectional study have a control group?
They differ from time series analysis, in which the behavior of one or more economic aggregates is traced through time. As briefly discussed earlier, multiple cross-sectional surveys are used to assess the changes in exposures and outcomes in a particular population. The result shows that the obese HIV participants (exposed) were two and a half times (2.5x) more likely to be sedentary than the non-obese participants (unexposed). If the OR for the dataset was equal to 1, then the exposure (obese) did not affect the outcome’s odds. In other words, the chance of being sedentary is the same in the exposed (obese) and the non-exposed (not obese) groups. Similarly, if the OR was less than 1, it implies that the exposed (obese) group, were less likely to be sedentary (outcome) compared to the non-obese group (unexposed) (Tenny & Hoffman, 2019).
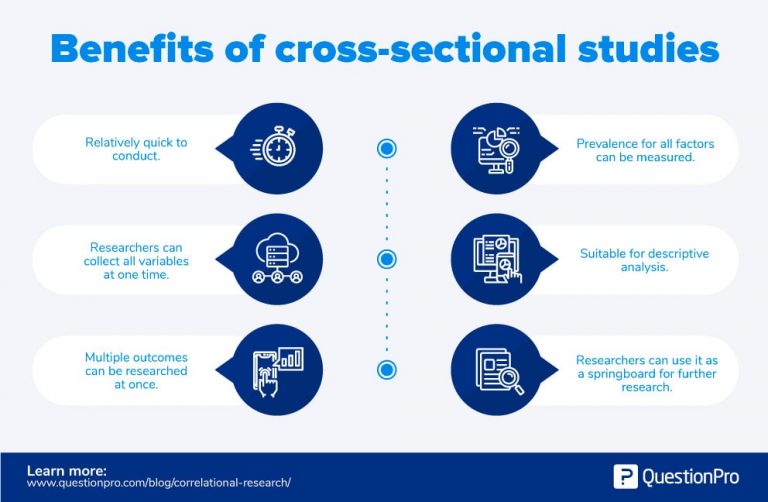
Cross-sectional vs longitudinal studies
To implement a cross-sectional study, you can rely on data assembled by another source or collect your own. There are several actions that could trigger this block including submitting a certain word or phrase, a SQL command or malformed data. Similar to the OR interpretation, if the RR was equal to 1, exposure did not prevent or harm the exposed and unexposed groups. In other words, being obese did not affect the activity level (sedentary versus not sedentary).
Types of Cross-Sectional Studies
This descriptive survey study investigates factors related to simulation educational satisfaction among nursing students. It’s a tool that allows researchers to collect data across a pre-defined subset or sample population at a single point in time. The information is typically about many individuals with multiple variables, such as gender and age.
Association between triglyceride glucose index and total bone mineral density: a cross-sectional study from NHANES ... - Nature.com
Association between triglyceride glucose index and total bone mineral density: a cross-sectional study from NHANES ....
Posted: Tue, 20 Feb 2024 08:00:00 GMT [source]
The analytical study designs of case-control, cohort and clinical trial will be discussed in detail in the next article in this series. Cross sectional study designs and case series form the lowest level of the aetiology hierarchy. In the cross sectional design, data concerning each subject is often recorded at one point in time.
Frequently asked questions about cross-sectional studies
The simulation design seemed to induce learners to flow into the simulation, which increased their simulation educational satisfaction because they performed well once they engaged in the simulation activity. The results of assessing the mediating effect of flow on the relationship between simulation design and simulation educational satisfaction of the participants were as follows (Table 4; Fig. 1). As a result of checking for multicollinearity among the independent variables to verify the assumptions for the regression analysis, the tolerance was 0.70, and the variance expansion factor was 1.43, indicating no multicollinearity. As a result of checking the autocorrelation of the dependent variable, the Durbin-Watson value was 2.06, which was close to 2, indicating that they were independent of each other. As nurses fulfill an essential role among healthcare workers, they experienced a particularly high occupational burden during the peak of coronavirus disease (COVID-19) pandemic [13]. In a multi-center cross-sectional online survey, among 395 healthcare workers, there were 42.28% and 56.2% were found to have depression, and anxiety during the COVID-19 pandemic, respectively [14].
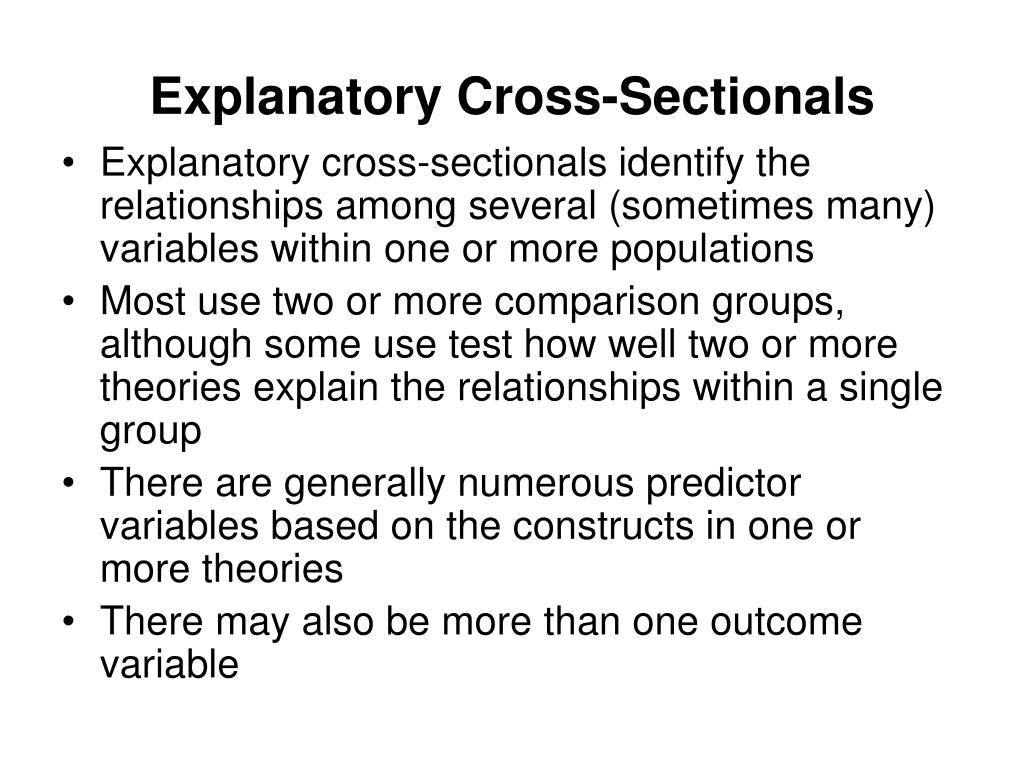
An extended stay in housing complexes that do not have a smoke-free policy puts residents at increased risk of exposure to SHS from individual flats, neighbouring flats, shared spaces, next buildings, and adjacent outdoor areas. Existing literature confirms that long-term exposure to SHS causes adverse health effects.39 However, further research is needed to validate the association between residents’ length of stay at home and their preference for smoke-free building policies. Among the respondents who wanted smoke-free housing, 44.9% preferred a smoke-free building policy, followed by a smoke-free common area policy (28.3%). This study found a positive association between BMI and poor mental health among nurses during the COVID-19 pandemic, particularly in those who were overweight or obesity. The findings could assist in developing interventions and help policy-makers establish appropriate strategies to support the mental health of frontline nurses, especially those who are overweight or obesity. A cross-sectional study (also known as a cross-sectional analysis, transverse study, prevalence study) is a type of observational study that analyzes data from a population, or a representative subset, at a specific point in time.
The Korean Accreditation Board of Nursing Education also allows simulation-based education to replace four credits of clinical practice credits in the third phase and up to six credits in the fourth phase [3]. A cross-sectional study will include several variables and sample groups, meaning it will collect data for all the different sample groups at once. However, in longitudinal studies, the same groups with similar variables can be observed repeatedly.
They can also develop and market a smaller phone to appeal to a more inclusive group of women. This research allows scholars and strategists to quickly collect cross-sectional data that helps in decision-making and offering products or services. Cross-sectional studies are popular because they have several benefits that are useful to researchers. Like any research design, cross-sectional studies have various benefits and drawbacks.
OR measures the association between exposure and outcome (see Table 1) and denotes the chances that an outcome happens with a specific exposure, compared to the chances of an outcome happening in the absence of the exposure (Szumilas, 2010). This information helps both clinicians and investigators determine if certain factors (i.e., clinical characteristics, medical history) are a risk for a particular outcome (i.e., disease, condition). Future studies or health policies can target methods to prevent or treat outcomes (i.e., disease, condition) identified in such studies.
In these studies, researchers study one group of people who have developed a particular condition and compare them to a sample without the disease. Longitudinal studies require more time and resources and can be less valid as participants might quit the study before the data has been fully collected. They can be beneficial for describing a population or “taking a snapshot” of a group of individuals at a single moment in time. Typically, these studies are used to measure the prevalence of health outcomes and describe the characteristics of a population.
While this study provides interesting insights, it is important to acknowledge its various limitations. First, since this study is cross-sectional, there is a concern for reverse causation, where mental health problems may contribute to increased BMI. Future studies with a longitudinal framework are warranted to address this issue.
These studies can map the prevailing variables that coexist at a specific point in time. For instance, cross-sectional data can reveal the buying preferences of a population at a given time and how they correlate with economic trends. Researchers prefer cross-sectional studies to find common points between variables. Still, they use longitudinal studies, due to their nature, to dissect the research from the cross-sectional studies for further research. This study type is also known as cross-sectional analysis, transverse study, or prevalence study. Although this research does not involve conducting experiments, researchers often use it to understand outcomes in the physical and social sciences and many business industries.
Cross-sectional studies cannot establish a cause-and-effect relationship or analyse behaviour over a period of time. To investigate cause and effect, you need to do a longitudinal study or an experimental study. Researchers in economics, psychology, medicine, epidemiology, and the other social sciences all make use of cross-sectional studies in their work. For example, epidemiologists who are interested in the current prevalence of a disease in a certain subset of the population might use a cross-sectional design to gather and analyse the relevant data. For investigators studying rare diseases or conditions, the cross-sectional design is not the best fit. Cross-sectional studies often draw samples from a large and heterogeneous study population (Wang & Cheng, 2020).
No comments:
Post a Comment